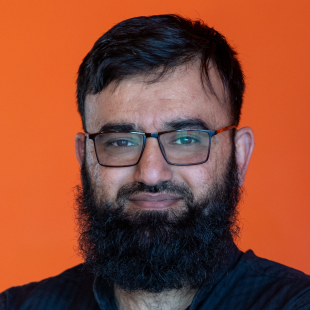
Umair Qudus
Data Science / Heinz Nixdorf Institute
Research Associate
Fact-checking over knowledge graphs Analyzing Linked Data—especially concerning its veracity Fake news detection
- E-Mail:
- uqudus@mail.uni-paderborn.de
- Phone:
- +49 5251 60-5190
- ORCID:
- 0000-0001-6714-8729
- Web:
- Homepage
- Homepage (Extern)
- Office Address:
-
Technologiepark 6
33100 Paderborn - Room:
- TP6.3.307
- Office hours:
Monday, 14:00 - 16:00
About Umair Qudus
I am a computer scientist. I graduated in Computer Science from the National University of Computing and Emerging Sciences, with a strong background in data science, semantic web technologies, and query processing. I worked as a research engineer at Kyung Hee University, South Korea. I am currently working as a Ph.D. candidate at Paderborn University, Germany, tackling the application of Knowledge Graphs such as Fact Checking using traversal, embedding, and NLP techniques to assess and improve the quality of large-scale knowledge graphs.
Furthermore, I have worked and learned from a variety of environments, always employing a hands-on approach to gathering and processing data. I apply machine learning solutions to real issues, creating value through proofs-of-concept, deployed models in production, or peer-reviewed scientific papers.
I have acted in R&D teams in both software engineering and researcher positions, have worked as a data scientist for multinational companies, and I am also very experienced in teaching machine learning to different audiences, from master's students to start-up entrepreneurs and even seasoned engineers.
I am always open to collaborating and learning from researchers and industry experts around the globe.
#FactChecking #KnowledgeGraphs #QueryOptimization #FederatedQueryProcessing
Research
Research Interests
I am mainly interested in:
- Fact-checking over knowledge graphs
- Analyzing Linked Data—especially concerning its veracity
- Fake news detection
Publications
Latest Publications
U. Qudus, M. Röder, D. Vollmers, A.-C. Ngonga Ngomo, in: Proceedings of the 33rd ACM International Conference on Information and Knowledge Management, ACM, 2024, pp. 3994–3999.
U. Qudus, M. Röder, F.L. Tatkeu Pekarou, A.A. Morim da Silva, A.-C. Ngonga Ngomo, in: M. Rospocher, Mehwish Alam (Eds.), EKAW 2024, 2024.
U. Qudus, M. Röder, S. Kirrane, A.-C. Ngonga Ngomo, in: T. R. Payne, V. Presutti, G. Qi, M. Poveda-Villalónt, G. Stoilos, L. Hollink, Z. Kaoudi, G. Cheng, J. Li (Eds.), The Semantic Web – ISWC 2023, Springer International Publishing, Cham, 2023, pp. 465–483.
U. Qudus, M. Röder, M. Saleem, A.-C. Ngonga Ngomo, in: U. Sattler, A. Hogan, M. Keet, V. Presutti (Eds.), The Semantic Web -- ISWC 2022, Springer International Publishing, Cham, n.d., pp. 462--480.
U. Qudus, M. Saleem, A.-C. Ngonga Ngomo, Y.-K. Lee, Semantic Web 12 (n.d.) 843–868.
Show all publications